Harnessing Data Science for Effective Lead Scoring Strategies

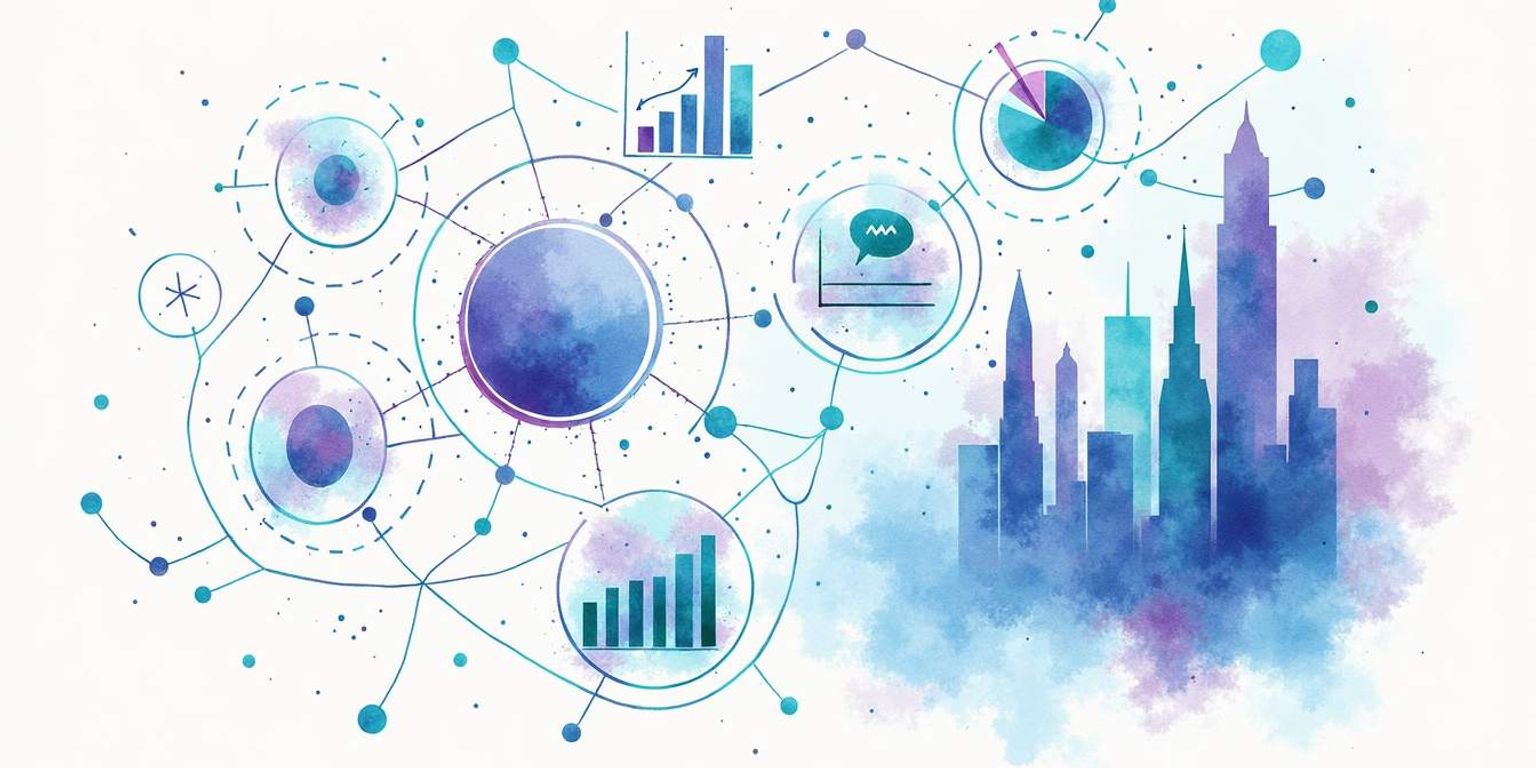
Harnessing Data Science for Effective Lead Scoring Strategies
Introduction to Lead Scoring
In the competitive landscape of sales and marketing, understanding which leads are most likely to convert is crucial. lead scoring is a systematic approach that helps businesses prioritize leads based on their potential to become customers. By leveraging data science, organizations can refine their lead scoring strategies, ensuring that their sales teams focus on the most promising prospects.
Data science provides the tools and methodologies necessary to analyze vast amounts of data, uncover patterns, and make informed predictions. This article explores how businesses can harness data science to develop effective lead scoring strategies, ultimately driving better sales outcomes.
Lead scoring typically involves assigning numerical values to leads based on various attributes, such as demographic information, engagement levels, and behavioral indicators. For instance, a lead who frequently visits a company’s website, downloads resources, and engages with social media content may receive a higher score than one who has merely filled out a contact form. This quantitative assessment allows sales teams to prioritize their outreach efforts, ensuring that they invest time and resources in leads that are more likely to convert into paying customers.
Moreover, the integration of machine learning algorithms into lead scoring systems can enhance accuracy and efficiency. By continuously analyzing historical data and adjusting scores based on real-time interactions, these algorithms can identify trends and shifts in customer behavior that might go unnoticed by traditional methods. As a result, businesses can stay agile and responsive to market changes, optimizing their sales strategies and improving overall conversion rates. This dynamic approach not only streamlines the sales process but also fosters a more personalized experience for potential customers, ultimately leading to stronger relationships and increased loyalty.
The Importance of Lead Scoring
Lead scoring is not just about identifying potential customers; it's about optimizing the sales process. By assigning scores to leads based on various criteria, businesses can streamline their efforts and allocate resources more efficiently.
Effective lead scoring can lead to increased conversion rates, shorter sales cycles, and improved overall sales performance. Understanding which leads are most likely to convert allows sales teams to tailor their approaches, enhancing customer engagement and satisfaction.
Understanding Lead Attributes
To implement a successful lead scoring system, it is essential to identify the attributes that contribute to a lead's likelihood of conversion. Common attributes include demographic information, behavioral data, and engagement levels. For instance, a lead's industry, company size, and geographic location can provide insights into their potential fit for a product or service.
Behavioral data, such as website visits, email opens, and social media interactions, can indicate a lead's interest level. By analyzing these attributes, businesses can create a more nuanced understanding of their leads, allowing for more accurate scoring.
Data-Driven Scoring Models
Data-driven scoring models utilize statistical techniques and machine learning algorithms to assign scores to leads based on historical data. These models can analyze past customer behavior and identify patterns that correlate with successful conversions.
For example, a model might reveal that leads who download a specific resource are more likely to convert than those who do not. By incorporating these insights into the lead scoring process, businesses can enhance their predictive accuracy and improve their overall sales strategies.
Implementing Data Science in Lead Scoring
Integrating data science into lead scoring involves several key steps, from data collection to model deployment. Each step plays a vital role in ensuring the effectiveness of the lead scoring system.
Data Collection and Integration
The first step in implementing a data-driven lead scoring system is to gather relevant data from various sources. This includes CRM systems, marketing automation tools, and external data providers. For instance, Clarify, a next-generation CRM, offers robust data integration capabilities, allowing businesses to consolidate information from multiple sources seamlessly.
Once the data is collected, it must be cleaned and organized to ensure accuracy. This process involves removing duplicates, filling in missing values, and standardizing formats. High-quality data is essential for building reliable scoring models.
Feature Engineering
Feature engineering is the process of selecting and transforming variables to improve model performance. This step is crucial in lead scoring, as the right features can significantly impact the accuracy of predictions.
For example, instead of using raw engagement metrics, businesses might create composite scores that combine multiple factors, such as frequency of website visits and time spent on key pages. By crafting meaningful features, organizations can enhance their lead scoring models and achieve better results.
Machine Learning Techniques for Lead Scoring
Machine learning offers powerful techniques that can be applied to lead scoring. By utilizing algorithms that learn from data, businesses can create dynamic scoring models that adapt to changing market conditions and customer behaviors.
Supervised Learning Models
Supervised learning models are trained on labeled datasets, where the outcome (conversion or non-conversion) is known. These models learn to associate specific features with the likelihood of conversion, allowing businesses to predict scores for new leads.
Common algorithms used in supervised learning for lead scoring include logistic regression, decision trees, and random forests. Each of these algorithms has its strengths and can be selected based on the specific needs of the organization.
Unsupervised Learning Approaches
Unsupervised learning techniques, such as clustering, can also be beneficial in lead scoring. These methods analyze data without predefined labels, helping businesses identify natural groupings among leads.
By clustering leads based on similar attributes or behaviors, organizations can tailor their marketing strategies to specific segments, enhancing engagement and conversion rates. This approach allows for a more personalized experience, which is increasingly important in today’s market.
Evaluating and Refining Lead Scoring Models
Once a lead scoring model is implemented, it is essential to continuously evaluate its performance. Regular assessments help identify areas for improvement and ensure that the model remains relevant as market conditions change.
Key Performance Indicators (KPIs)
Establishing KPIs is crucial for measuring the effectiveness of lead scoring models. Common KPIs include conversion rates, the accuracy of predictions, and the overall impact on sales performance. By tracking these metrics, businesses can gain insights into the effectiveness of their lead scoring strategies.
For example, if the conversion rate for high-scoring leads is significantly higher than for lower-scoring leads, it indicates that the model is functioning effectively. Conversely, if there is little difference in conversion rates, it may be time to revisit the scoring criteria and model parameters.
Iterative Refinement
Data science is an iterative process, and refining lead scoring models is no exception. Continuous learning from new data and feedback is essential for maintaining the accuracy and relevance of the scoring system.
Organizations should regularly update their models with fresh data, reassess feature importance, and experiment with different algorithms. This iterative approach ensures that businesses remain agile and responsive to changes in customer behavior and market dynamics.
The Role of CRMs in Lead Scoring
Customer Relationship Management (CRM) systems play a pivotal role in lead scoring strategies. A robust CRM, like Clarify, can provide the necessary infrastructure for collecting, storing, and analyzing lead data.
Centralized Data Management
CRMs centralize lead information, making it easier for sales teams to access and manage data. This centralized approach is essential for effective lead scoring, as it allows for a comprehensive view of each lead's interactions and behaviors.
With a CRM that integrates seamlessly with marketing automation tools, businesses can gather data from various touchpoints, ensuring that their lead scoring models are based on a complete dataset. This holistic view enhances the accuracy of predictions and improves overall sales strategies.
Automation of Lead Scoring Processes
Automation is another significant advantage of using a CRM for lead scoring. By automating the scoring process, organizations can save time and reduce manual errors. Automated lead scoring ensures that leads are assigned scores in real-time, allowing sales teams to act quickly on high-potential prospects.
Furthermore, advanced CRMs can incorporate machine learning algorithms directly into their systems, enabling businesses to leverage data science without needing extensive technical expertise. This democratization of data science empowers more organizations to adopt sophisticated lead scoring strategies.
Case Studies: Successful Lead Scoring Implementations
Examining real-world examples of successful lead scoring implementations can provide valuable insights into best practices and strategies. These case studies highlight how organizations have effectively harnessed data science to enhance their lead scoring processes.
Case Study 1: E-Commerce Company
An e-commerce company implemented a data-driven lead scoring model to improve its email marketing campaigns. By analyzing customer behavior, such as purchase history and browsing patterns, the company developed a scoring system that prioritized leads based on their likelihood to make a purchase.
The results were impressive: the company saw a 30% increase in conversion rates among high-scoring leads. By focusing their marketing efforts on these leads, they were able to optimize their resources and drive significant revenue growth.
Case Study 2: B2B SaaS Provider
A B2B SaaS provider utilized machine learning algorithms to refine its lead scoring model. By integrating data from its CRM and marketing automation tools, the company developed a dynamic scoring system that adapted to changing customer behaviors.
This approach led to a 25% reduction in the sales cycle, as sales teams could prioritize high-scoring leads more effectively. The organization also reported an increase in customer satisfaction, as they were able to engage prospects with tailored messaging that resonated with their needs.
Conclusion
Harnessing data science for effective lead scoring strategies is no longer a luxury; it is a necessity in today's competitive marketplace. By leveraging data-driven insights, organizations can optimize their sales processes, improve conversion rates, and drive revenue growth.
As businesses continue to embrace advanced technologies, the integration of data science into lead scoring will become increasingly sophisticated. CRMs like Clarify are at the forefront of this evolution, providing the tools and capabilities necessary for organizations to thrive.
In conclusion, adopting a data-driven approach to lead scoring not only enhances sales performance but also fosters a deeper understanding of customer needs. As organizations refine their strategies and embrace the power of data science, they will be well-positioned to succeed in an ever-changing landscape.
Take Your Lead Scoring to the Next Level with Clarify
Ready to transform your lead scoring with the power of data science? Clarify is your partner in this journey, offering a cutting-edge CRM platform that integrates seamlessly with your sales and marketing strategies. With our AI-driven insights and automation, you can focus on nurturing high-potential leads and driving conversions. Don't miss out on the opportunity to elevate your business. Request access to Clarify today and experience the future of customer relationship management.
Get our newsletter
Subscribe for weekly essays on GTM, RevTech, and Clarify’s latest updates.
Thanks for subscribing! We'll send only our best stuff. Your information will not be shared and you can unsubscribe at any time.